Siew Li, Linda Tan
Postgraduate:
Main Appointment:
Joint Appointments:
Research Fields:
Research Areas:
Research Fields:
- STEMM – Science, Technology, Engineering, Mathematics, Medical Sciences
Research Keywords:
- Bayesian Inference
- Variational Approximation
- Hierarchical Models
- Stochastic Optimization
- Network Modeling
Current Appointments:
Brief Description of Research:
Driven by technology, the trend towards collecting large data has resulted in the need for more flexible models to derive meaningful and actionable insights from such data and fast computational methods for fitting them. My research addresses these needs by investigating ways of speeding up and improving the accuracy of the derivation of classical and Bayesian statistical inference from complex models applied to large data sets. In particular, Bayesian methods offer a flexible and principled approach to inference and prediction that allows one to synthesize data from various sources while quantifying for uncertainty. However, it is often challenging to obtain Bayesian inference from complex models due to the computationally intensive characteristic of Markov Chain Monte Carlo (MCMC) methods. My research studies some important alternatives such as variational approximation and stochastic optimization, as well as ways to improve the rate of convergence of MCMC methods and the EM algorithm for classical statistical inference, by studying the close links between these methods.
Total Number of Publications:
Five Representative Publications:
Tan, L. S. L. (2022). Efficient data augmentation techniques for some classes of state space models. Statistical Science (in press).
Tan, L. S. L. (2021). Use of model reparametrization to improve variational Bayes. Journal of the Royal Statistical Society: Series B (Statistical Methodology), 83 (1), 30-57. https://doi.org/10.1111/rssb.12399
Tan, L. S. L. and Friel, N. (2020). Bayesian variational inference for exponential random graph models. Journal of Computational and Graphical Statistics, 29 (4), 910-928. https://doi.org/10.1080/10618600.2020.1740714
Tan, L. S. L., Jasra, A., De Iorio, M. and Ebbels, T. M. D. (2017). Bayesian inference for multiple Gaussian graphical models with application to metabolic association networks. Annals of Applied Statistics, 11 (4), 2222–2251. https://doi.org/10.1214/17-AOAS1076
Tan, L. S. L., Chan, A. H. and Zheng, T. (2016). Topic-adjusted visibility metric for scientific articles. Annals of Applied Statistics, 10 (1), 1–31. https://doi.org/10.1214/15-AOAS887
My Research Videos:
Top 5 Publications:
- Lorem ipsum dolor sit amet
- Lorem ipsum dolor sit amet
- Lorem ipsum dolor sit amet
- Lorem ipsum dolor sit amet
- Lorem ipsum dolor sit amet
Journals Published:
- Lorem ipsum dolor sit amet
- Lorem ipsum dolor sit amet
- Lorem ipsum dolor sit amet
- Lorem ipsum dolor sit amet
- Lorem ipsum dolor sit amet
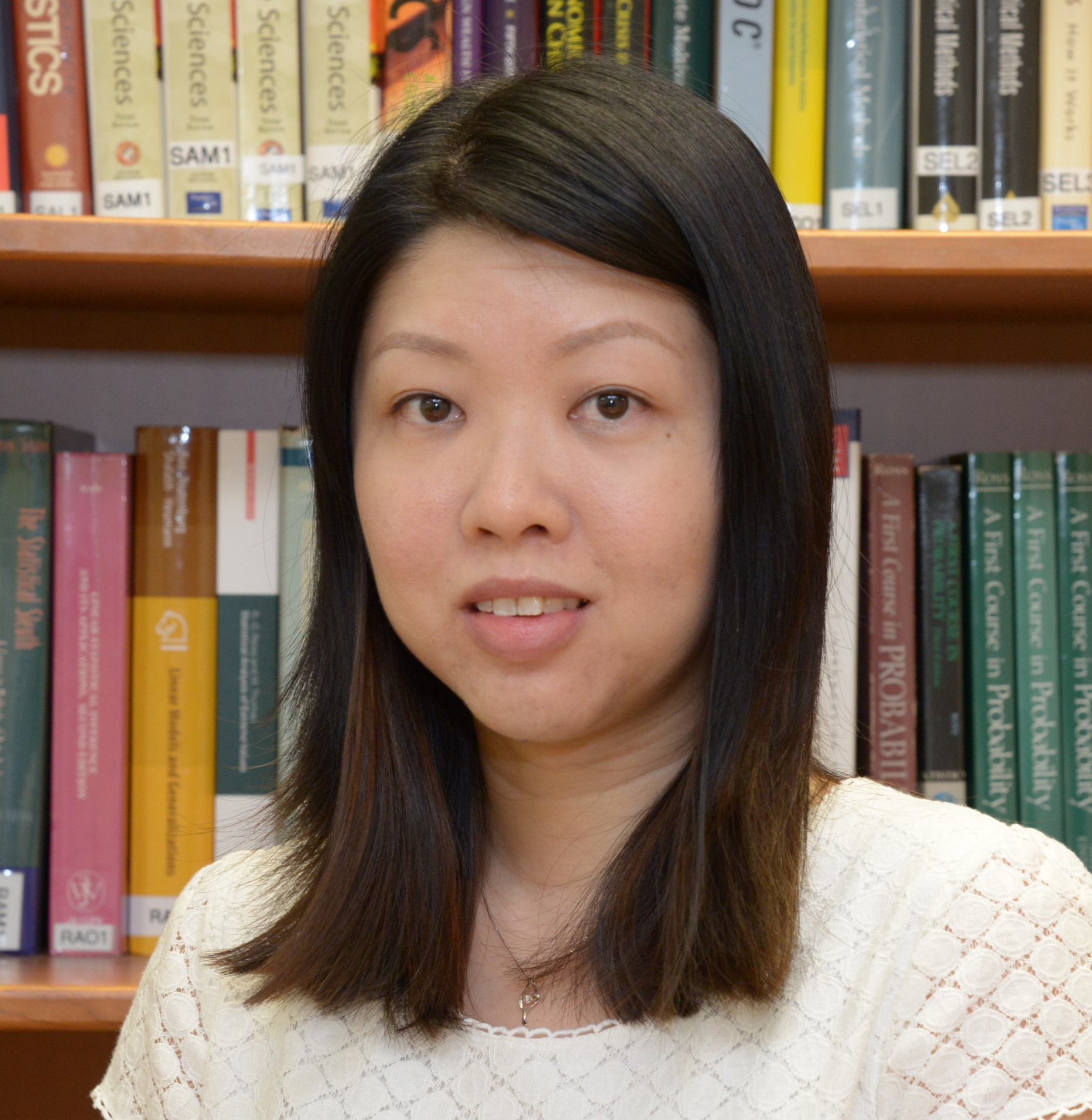
Appointments
Education
Research Areas
- Bayesian Inference
- Variational Approximation
- Hierarchical Models
- Stochastic Optimization
- Network Modeling
Research Description
Driven by technology, the trend towards collecting large data has resulted in the need for more flexible models to derive meaningful and actionable insights from such data and fast computational methods for fitting them. My research addresses these needs by investigating ways of speeding up and improving the accuracy of the derivation of classical and Bayesian statistical inference from complex models applied to large data sets. In particular, Bayesian methods offer a flexible and principled approach to inference and prediction that allows one to synthesize data from various sources while quantifying for uncertainty. However, it is often challenging to obtain Bayesian inference from complex models due to the computationally intensive characteristic of Markov Chain Monte Carlo (MCMC) methods. My research studies some important alternatives such as variational approximation and stochastic optimization, as well as ways to improve the rate of convergence of MCMC methods and the EM algorithm for classical statistical inference, by studying the close links between these methods.
Research Videos
Selected Publications
Tan, L. S. L. (2022). Efficient data augmentation techniques for some classes of state space models. Statistical Science (in press).
Tan, L. S. L. (2021). Use of model reparametrization to improve variational Bayes. Journal of the Royal Statistical Society: Series B (Statistical Methodology), 83 (1), 30-57. https://doi.org/10.1111/rssb.12399
Tan, L. S. L. and Friel, N. (2020). Bayesian variational inference for exponential random graph models. Journal of Computational and Graphical Statistics, 29 (4), 910-928. https://doi.org/10.1080/10618600.2020.1740714
Tan, L. S. L., Jasra, A., De Iorio, M. and Ebbels, T. M. D. (2017). Bayesian inference for multiple Gaussian graphical models with application to metabolic association networks. Annals of Applied Statistics, 11 (4), 2222–2251. https://doi.org/10.1214/17-AOAS1076
Tan, L. S. L., Chan, A. H. and Zheng, T. (2016). Topic-adjusted visibility metric for scientific articles. Annals of Applied Statistics, 10 (1), 1–31. https://doi.org/10.1214/15-AOAS887